Leveraging an AI-powered Salesforce CRM Will Require Better Data Management
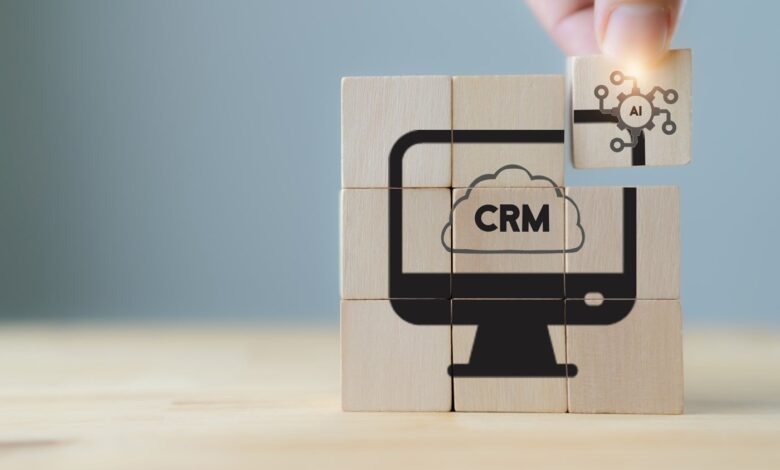
[ad_1]
Jack Wagnon has been a customer relationship management consultant for over a decade. Now, as global head of Rimini Street’s Salesforce practice, he thinks artificial intelligence is about to deliver on “the original promise of customer relationship management” for APAC enterprises — a true 360-degree view of the customer.
This will not be without struggle. As Salesforce rolls out AI and organisations seek to automate and capitalise on the right customer information at the right time, Wagnon said businesses will need to work hard to master their data and get AI use cases budgeted for and implemented.
Software Spotlight: SalesforceA leader in the CRM market, Salesforce is an all-rounded cloud-based platform for managing sales, marketing and customer interactions. For more information, check out our in-depth Salesforce review. |
4 key steps to data management mastery for Salesforce
Rimini Street, a Salesforce partner, argues the “playbook” comes down to four key steps:
- Designing and building a master data management strategy.
- Looking hard at data integrations and designing for scale.
- Start budget planning for AI feature enablement.
- Create a delivery roadmap for AI use cases.
1. Design and build a master data management strategy
Salesforce research indicates the average number of apps across organisations’ digital estates is now 991. AI adoption is further tangling this “API sprawl.” Further, 95% of organisations say integration is impeding AI adoption, with only 28% of apps actually connected. (Figure A).
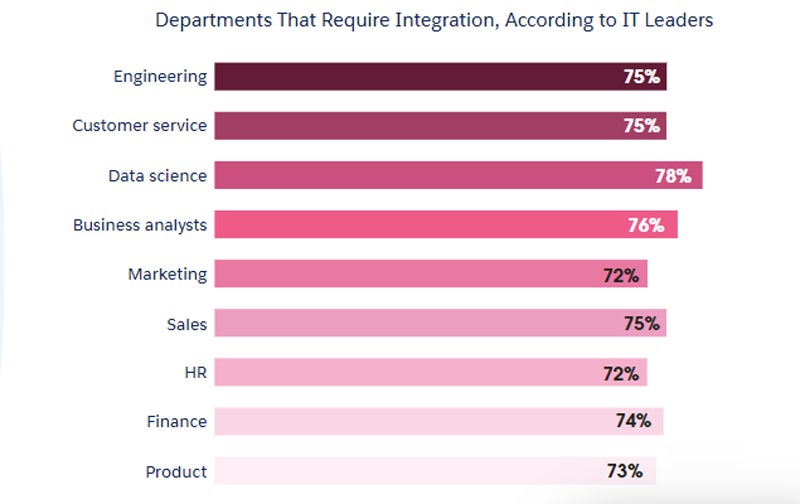
Wagnon said data fragmentation and unstructured data is a significant challenge for enterprises. He said creating structured data environments is not easy because it requires tackling multiple moving parts, like putting in place system reconfiguration agreements on data collected.
Wagnon recommends a master data management strategy involving a structured data framework and tight integrations.
“Once you’ve ingested it, then it becomes understanding the good versus the bad; the risk with AI-driven automation is you trigger an action that’s wrong,” Wagnon said.
Top-down C-suite executive support critical to CRM data strategy
Master data management strategy success depends on top-down executive buy-in and support, according to Wagnon. He said the most likely source of failure is relying on “leadership in the middle,” who may fight a losing battle against the weight of an existing corporate structure.
SEE: Australian cyber security pros also face struggles with engaging executives.
“Without executive buy-in, which translates to a funded mandate, getting to a master data management strategy becomes next to impossible,” Wagnon said. “If you don’t have that support, even strong middle leadership will struggle seeing this happen in a reasonable amount of time.”
2. Examine data integrations and design for scale across ERP
Rimini Street’s business has been built on providing third-party support to enterprises using Oracle and SAP ERP systems. Wagnon said that, based on this experience, understanding of the enterprise resource planning stack is important in delivering on the full promise of an AI-powered CRM system.
Wagnon said a range of broader ERP stack functions, like supply chain or accounting, generate customer based data. Ignoring these connections when building CRM tools and capabilities means creating more siloed data or minimising the ability to scale “without breaking,” he said.
The ability to see the larger playing field across the ERP stack can support a lower total cost of ownership and improve day-to-day management efficiency.
“It’s not just financial costs, but also sheer complexity,” said Wagnon. “It reduces the challenge of administering a system when it scales well.”
3. Start budget planning for AI feature enablement
Budget planning for AI-feature enablement starts with educating internal stakeholders, in particular the CFO. Wagnon said if the CFO does not understand the benefit of moving dollars from day-to-day operations to innovation and expansion, getting funded becomes difficult.
“The CFO is your best friend when it comes to building consensus internally,” Wagnon said.
Business budgets are approximately 90% focused on day-to-day operations and about 10% on business transformation. Rimini Street looks to create savings in some areas and reinvest in others to turn this into a 60/40% split between operations and transformation spending.
4. Create a roadmap for the delivery of AI use cases
Setting aside enough budget for AI-related innovation is as much about creating a roadmap of the capabilities that need to be prioritised. Wagnon said the 24 months of dollar allocation required for investing in this innovation goes together with a strong roadmap of prioritisations.
SEE: How Australia’s Zetaris is serving data up for AI with federated data lakes.
“This roadmap is probably the second most difficult thing after the structured data challenge, because it involves getting people to agree that, out of all the things that AI could do for us, we want to do this first, then second and then third,” Wagnon said. “If you develop the consensus, you can work with your technology delivery partners and say, ‘We think this is the business function that will give us the greatest bang for our buck, please help me build it.’
“You have alignment between the business and technology delivery.”
Pragmatic steps required to draw value from AI-powered CRMs
Wagnon said there are some key use cases emerging for AI-powered CRMs. These include the automation of customer service responses to improve customer experiences and improving lead generation through automated analysis and actions based on customer behaviour.
As a “nuts and bolts” guy, Wagnon said using AI to crawl systems for optimisations was another key use case; automated AI-driven analysis can identify where it would be valuable to fix a system in cases where, for example, data is not being inputted or captured correctly.
But to truly leverage AI, organisations may need to get some “big rock” items out of the way.
“We’ve built our Salesforce practice around pragmatism, about what to do to realise this benefit,” Wagnon said. “And we spent a lot of time through our strategic advisory services having candid conversations about the order of priorities and, if you really want to see the value, the path to get there.
“A lot of that journey is not very sexy. The AI ending is alluring, but the work to get there is about structured data, integration standards, funding, and CIO, CFO and CEO-authorised mandates. Because we’re talking major systemic change within the computing environment.”
[ad_2]
Source Credit